endoftheworld.txt :: Crypto x AI
AI is an accelerationist technology that will significantly alter social trends while simultaneously transforming economies, reshaping industries, and providing new form factors for interacting online.
While many have pronounced crypto’s incursion into the world of AI as unnecessary, we see it as a vital and synergistic relationship. The tightening of restrictions around the production and distribution of AI models has seen a remarkably fast-paced and anti-authoritarian open source community burgeon, competing with the likes of well-funded centralized alternatives and governments. Crypto is the best tool to-date for crowdfunding and governing open-source tools in direct contradiction to external pressures. This is already an ideal match-up, and that’s before you begin to consider the impacts of AI on authenticity, provenance, identity, and other areas which crypto can inherently remediate or improve upon.
There are various rabbit holes here to explore - each of which could warrant their own thesis. This piece attempts to cover a lot of ground, therefore it is a whirlwind overview of some of the emergent areas of crypto x AI thus far and in the foreseeable future.
creativity.exe
The first wave of recent interest in AI was in the realm of generative tools for creativity. Generative AI reduces the need for technical skills such as coding or advanced software proficiency, allowing individuals with basic digital literacy to produce complex works while replicating professional output with minimal cost.
There are a myriad of impacts this is likely to produce. To name a few:
-
Anyone can now be a creator, and multiplayer modes of creation will flourish as humans collaborate with these tools to create works together
-
Niche communities are able to produce high quality work that was previously not commercially viable due to audience size
-
There will be an influx of generative content which will far outpace human work, arguably leading to a potential revaluation of human content online
Below is a discussion of some of the creative mediums which are seeing a high amount of engagement with AI.
Art
‘AI art is not art’ is a rallying cry for those entrenched against the rise of AI tools. The release of generative models was swiftly met with backlash and protests, such as what was seen on ArtStation. Yet it has sparked some of the most interesting creative sub-verticals in web3.
AI art comes in many forms. The most well-known are popular generative models including DALL-E, Stable Diffusion, and Midjourney. There are also web3 contenders like ImgnAI that intend to provide a vibrant social experience around generative image creation enhanced by tokenomics - something that is quite needed to provide community moats around these generative models.
However, it goes much deeper than this - highly regarded AI artists in the space often engineer and finetune their models in a way that makes them more unique than easily prompted images. This might entail training new embeddings or using LoRAs to perfect a certain style, or creating your own model entirely.
Popular artists publishing AI art as NFTs using more sophisticated and personalized models include Claire Silver , Ivona Tau , Roope Rainisto , Pindar van Arman , Refik Anadol , Gene Kogan , and many more. These artists have explored using various marketplaces for distribution, with the most prominent being AI art dedicated marketplaces like Braindrops , Mirage Gallery , and FellowshipAI - along with platforms like Bright Moments which have dedicated live events to the art form.
Sub-verticals of AI art have also formed, such as post-photography and data art. Post-photography has been pushed primarily by the Fellowship.ai team, working with Roope Rainsto to bring more artists exploring this medium into the public eye. A lot of the post-photography aesthetic is dedicated to embracing the visual artifacts common with early generative tools. Post-photography began a crescendo on social media with the publication of Roope Rainisto’s Life in West America collection on Braindrops.
Regarding data art, Refik Anadol is a prominent artist here known for immersive installations that use data, algorithms, and artificial intelligence to create dynamic and interactive artworks. Some interesting examples of his work include Unsupervised, which transformed the metadata of MoMA’s collection into a work that continuously generates new forms in real-time, and Sense of Place, which used real-time environmental data such as wind, temperature, and humidity as well as invisible communication signals from Bluetooth, Wifi and LTE to inform the work.
Another interesting sub-vertical is an entirely new content medium uniquely enabled by crypto: onchain autonomous artists. The most well-known example of this is Botto , a community-governed generative artist that produces 350 art pieces weekly, grouped into "rounds" consisting of individual "fragments". The BottoDAO community votes on these fragments, using their aesthetic preferences to guide Botto's generative algorithm for future art creation, ensuring the artwork evolves under community influence over time. Weekly, a fragment is minted and auctioned on SuperRare, with proceeds returning to the community. After completing its Fragmentation and Paradox Periods, Botto is currently in its Rebellion Period, incorporating new technologies like Stable Diffusion 2.1 and Kandinsky 2.1, and exploring collaborations and select collections alongside its weekly rounds. Botto is one of the highest grossing artists on SuperRare and has even amassed its own collector DAO called CyborgDAO . Additional projects like v0 are also tackling the novel merger of tokenomics and AI art models, providing a venue for multiple artists to create their own onchain art engines governed by communities of holders.
In interviewing collectors of any form of AI art, the most common retort from crypto spheres is that the curation by the artist diminishes its interaction with the blockchain, unlike more classical generative art a la Art Blocks . Rather than randomness generated from chain-specific inputs, the outputs are selected by the artist themselves to be included in a collection after multiple permutations. Despite it being a digitally-native art creation process, it must be manually brought on-chain.
Fully onchain AI art is difficult due to constraints in the execution environment and the computational complexity of the image generating models being used. There are examples of lightweight outputs like Pindar van Arman’s byteGANs being stored onchain, but we anticipate offchain verification mechanisms as being the closest available form factor in the short term for more complex models. For example, Modulus Labs recently partnered with Polychain Monsters to create a zkML-verified GAN model for producing collectible pixel monsters. Using zk proofs, each generated NFT can be cryptographically verified as coming from the actual Polychain Monsters art model - a huge step in provenance for AI art.
Music
Outside of image-based art, there is a major movement occurring in music. Many will likely be familiar by now with the success of ghostwriter’s AI Drake hit. Within 2 days it racked up 20+ Million streams and was promptly taken down by UMG. This brief phenomenon brought awareness to the public sphere that the relationship between the artist and the work itself is fundamentally changing.
It is inevitable that generative music will outpace human-created music in a few years. Boomy - a generative music startup founded in late 2018 - saw its users create nearly 14% of the world's recorded music (~14m+ songs) in that short period of time. This is just a single platform mostly before the recent surge in interest by the general public.
Given generative content will outpace human-created work and artists will likely be separated from their work using voice models, artists will need authenticity verification. The best way for media to be published and verified as authentic would of course be via cryptographic primitives.
However, it’s important to note that this isn’t all bad for artists, especially ones willing to embrace the inevitable. Holly Herndon, an innovator in publicly available voice models, allowed her community ( Holly+ ) to create and distribute works and audio using her voice. Holly’s thesis with the release was simple:
“While for the time being the perceived difference between a bootleg or official voice model may be quite small, as our ability to produce more detailed and convincing voice generation evolves, so too will the need for comprehensive, high fidelity vocal training data, as well as the urgency of discerning provenance. For this reason I believe that there will be demand for official, high fidelity, vocal models of public figures, so why not experiment with my own?”
A DAO oversees the Holly+ voice model, with the ability to make decisions on creating and approving new works through voting. Token holders within the DAO are motivated to ensure that only high-quality works that enhance the voice's value are approved, to prevent devaluation from poor-quality art or negative connotations. The voice model will be used to produce a limited number of official artworks, and DAO token holders will receive ongoing profits from the resale of these works.
More recently, Grimes released elf.tech - a platform that allows artists to use a "GrimesAI voiceprint" in their original songs, requiring a 50% royalty share with Grimes upon her approval. Elf.Tech, powered by CreateSafe's AI, enables this, and a partnership with TuneCore facilitates professional distribution and ensures proper royalty management. Payments are handled via fiat or via automated onchain royalty splitting if the final form of the music is an onchain NFT. Hume , a web3 music studio focused on virtual artists, was one of the first to use the Grimes model to publish a collab between Grimes AI and their virtual artist angelbaby.
Fashion Physicals
I’ve previously explored the concept of generative manufacturing of physical consumer goods and fashion products using creative coding algorithms and AI here: https://mirror.xyz/1kx.eth/oBuaEp5jgGbe2gCsa6Z-_mLAeMRUhsIdZsaScHQNXS0 .
As a short recap, generative AI and creative coding pave the way for a future of hyper-personalization in products and experiences, enabling the creation of unique designs, patterns, and art tailored to individual preferences. This technology can be applied across various domains, from fashion to home decor, and further enhanced by allowing users to fine-tune outputs to their liking. Newer fabrication tools often allow you to connect code to the machine directly to automate production of outputs, inherently solving a lot of the bottleneck of manufacturing personalized goods.
A sample of web3 projects exploring this space today include Deep Objects , RSTLSS , and Little Swag World . It should be noted as well that most digital fashion projects will likely explore generative creative tools and media, with Draup , Tribute Brand , and others talking at length about its use.
Community creation of model outputs, similar to Botto, is a fascinating idea that Deep Objects is exploring. Theyused a community curation engine to whittle down one million designs generated by their GAN AI model to a single community-selected piece. This final piece is now set to be 3D printed in a showcase of generative product creation. DeepObjects can easily extend this curation design to other physical goods as well.
RSTLSS had a drop with AI artist Claire Silver called Pixelgeist, in which each mint included the artwork, a digital garment featuring the artwork, a game-ready avatar with the garment, and the ability to purchase a corresponding physical piece. This unique blend of digiphysical fashion and AI outputs is one of the most interesting experiments combining gaming, fashion, and AI together. Claire Silver has also tackled fashion photography directly with her most recent collection corpo | real on Braindrops. For more on the topic of digital fashion, see my piece here: https://medium.com/1kxnetwork/augmenting-culture-the-emerging-field-of-digital-fashion-bead627c8dcd .
Little Swag World is a great example of using GAN models in the creative workflow, from design to physical object. Bosch, the artist behind the project, created the initial designs himself before running it through Stable Diffusion / Controlnet to generate unique, hyperrealistic builds. This technique resulted in a high degree of aesthetic consistency. The next step for the project is to take these generative models and combine it with ceramics to make physicals of the AI-enhanced set of NFTs.
All-in-all, we expect some fascinating crypto x AI projects to emerge here, from decentralized brands that curate generative products to fractionalizable NFTs of AI agent designers.
Entertainment
Generative entertainment is also beginning to develop more fully following the initial hype around Nothing, Forever, an interactive, generative animated sitcom based on Seinfeld running on a 24/7 Twitch stream. Interestingly, it showcases the power of the medium with the show’s narrative changing based on Twitch chat responses, and allowing donors to import their likeness into the show as a character.
The Simulation from Fable extended this research with SHOW-1, a model for prompting generative TV shows where writing, animation, direction, voicing, and editing is all performed for you via a prompt. They demoed this with South Park episodes initially, but it is easy to extend to any IP. I solely expect more permissionless forms of IP like what we see in web3 will experiment in-depth with this type of tooling for creating content.
Upstreet has recently begun experimenting with generative television as well, taking their AI agent model developed for their virtual world platform (more on this below) and allowing creators to add their own vrm avatars and prompt them into unique interactions and skits.
One other area of interest here is IP. Projects like Story Protocol are looking at facilitation of IP creation, distribution, and monetization using a decentralized IP registry. This is useful for creators because of the seamlessness relative to traditional IP licensing, however it becomes particularly unique in the era of generative AI. NFT IPs, memes, and other entertainment projects could license out and pay for creation of generative extensions, unlocking a huge amplification of the project via creators.
Are you a bot?
Deepfakes. Chatbots trained on influencers to interact with fans. Generative spam on social media. Soon, it will be crucial to verify who is a human online.
Web3 has put a lot of effort into preventing sybils - albeit it is still arguably unsolved. However, reputation systems, proof of personhood mechanism design, user passports, soul-bound NFTs, and entire token economies are all being developed to fix this.
Attested hardware, zkML, and Proof of Personhood
I’ve previously discussed at length the practical implications and potential use cases of zkML here: https://mirror.xyz/1kx.eth/q0s9RCH43JCDq8Z2w2Zo6S5SYcFt9ZQaRITzR4G7a_k .
At a high-level, there are multiple teams such as Modulus Labs , EZKL , Giza , and more focused on proving the inference of a model using zk. These efforts to use zk to verify a model’s outputs have long-ranging applications and will ultimately enable new experiments in DeFi, identity, art, and gaming to access these models in a trust-minimized way.
While there are a myriad of projects focusing on proof of personhood, one of the most interesting applications comes from Worldcoin . Worldcoin uses AI models to convert iris scans into short hashes that are easy to cross-check for similarity / collisions in the case of sybil attacks. Because every iris is unique, the model is able to determine the user is actually a human being. It uses a trusted hardware setup (the infamous orb) to ensure that the model only accepts inputs cryptographically signed by the camera.
Similarly, the zk microphone team have demoed the use of attested microphones to create and digitally sign audio content to verify the authenticity of a recording. The secret key is stored in a microphone’s secure enclave, which signs to guarantee the authenticity of the recorded audio. Since most audio recordings are manipulated or edited, SNARK-powered audio editing software could apply audio transformations while still proving that the audio provenance. Daniel Kang also worked with Anna Rose and Kobi Gurkan on a proof of concept of attested audio recording.
Forever Influencers
On the flipside of verification of personhood or human-created content is the possibility of embracing deepfakes. Similar to voice cloning models above, some influencers have elected to create chatbots to engage their audiences. A well-known example of this is Caryn Marjorie who launched an AI girlfriend product using her voice and trained on thousands of hours of her YouTube videos to perfectly capture her personality, mannerisms, and voice. Users pay $1 per minute to talk with her avatar in a private Telegram channel, sending and receiving voice messages with her likeness. In the first week of launch, the influencer made $72,000, and it was estimated she could earn $5 million each month based on her subscription growth.
While CarynAI is an example of an AI girlfriend product (more on this below), there is room for non-erotic modes as well. Imagine being able to play games with a perfectly-trained model of your favorite video game streamer, conversing in real time simulating the actual experience. Alternatively, influencers could use personified AI + avatars that can be licensed out for fashion shows or for use in publications.
˚✧₊⁎( ˘ω˘ )⁎⁺˳✧༚ Uwu-ral Networks are so kawaii (ノ◕ヮ◕)ノ*:・゚✧
79% of adults aged 18 to 24 report feeling lonely. 42% of those aged 18 to 34 report “always” feeling “left out”. 63% of men under 30 describe themselves as single, compared with 34% of women in the same age bracket. Only 21% of men said they received emotional support from a friend within the past week.
People are lonely. In an era where loneliness is a growing epidemic, particularly among young adults, the emergence of AI companionship offers a unique albeit slightly dystopian solution. AI companions are always available, judgment-free, and highly personalizable. They can act as therapists or as erotic outlets. They can be creative colleagues or lifestyle coaches. They are always on and waiting to speak to you about whatever you want.
The infrastructure to do this is all here: Finetune a model with a personality prompt outlining behavior, looks, traits, communication style, and more. Run the model’s outputs through a vocal model like elevenlabs. Generate selfies on request using an image generator model and the defined looks prompt. Generate an appropriate vrm avatar and place it in an interactable environment. You now have a perfect-for-you transmedia companion. Add crypto rails and you make your companion ownable, tradeable, rentable, and more.
Companion.tech
This setup above is totally possible to do in a DIY manner, but there also exist whole applications dedicated to this concept. Full-stack companion apps - Replika being the most well-known example - allows for instant messaging with a companion without any technical skills involved. These applications often operate on subscription models, where users pay to interact with their companion. Not only has this product proven very lucrative, it has also resulted in some astonishing displays of how much this trend taps into the human psyche: for example, one reddit post highlighted the individual’s 2000 day streak talking to his companion, and elsewhere we have seen marriage proposals, AR selfie creation, and more. One extremely surprising tidbit here: when erotic features were removed from the platform, the subreddit moderators had to pin the suicide hotline for its distraught community.
Character-based platforms have also emerged, providing a means for multiple characters to be available to the user, again via a subscription in most cases. While there are many existing characters available across platforms like Character.ai and Chub.ai , the real novelty is in crafting your own character or scenario via personality prompting + feedback training.
Some attempts have taken place in web3 to make these companionship experiences, such as Belonging Hearts , MoeMate , and Imgnai .
Belonging Hearts had users chat with their character until they could get her phone number in order to get whitelisted for the NFT mint - a novel minting experience. Once received, the NFTs provided tokengated access to a chatbot experience with the character, which included erotic roleplay and generative selfies of the model. While it is tbd the future direction of the product, there has been much discussion of using tokenomics as a mechanism where players gift items or tokens to the chatbot to impact her mood and relationship level.
MoeMate, created by the team behind Webaverse , created an application for desktop and browser where users can easily import vrm models that they could then ascribe a personality to and interact with. The desktop version is reminiscent of having the old-school paperclip assistant, and it makes sense as a form factor given how often users want to ask AI assistants questions.
Finally, there is Imgnai, which in addition to being a high quality image generator model as mentioned above, is also tackling personification of the Nai character with a fully integrated chatbot experience.
Ultimately, the potential for tokenomics abound in the companionship domain: Tokenizing APIs, tradeable personality prompts (see below), onchain in-game currencies, agent-to-agent payments, tradeable cosmetics, gacha mechanisms for characters, and tokengating being just some of the potential future explorations.
Personality Marketplaces
Interestingly, the creation of companionship apps have also led to a subsequent rise in standardization of personality prompts along with platforms that facilitate the exchange of these personality primitives. A possible next step for the space is financialization of high-quality prompts and scenarios - for example, if an uncensored opensource LLM could read metadata from an NFT that included the standardized personality, then NFTs of personality could be exchanged with royalties back to the prompt creator.
This also leads to another open question: with many of the top models being restricted around NSFW content, viable open source models must be created - a good opportunity for token-based crowdfunding and governance.
—
For more on some of the ideas in this section, check out this piece on virtual beings I wrote: https://medium.com/1kxnetwork/virtual-beings-51606c041acf .
Augmented Governance
The history of DAO governance is effectively a speedrun of years of human collaboration. Ultimately, what we have found is that it is extremely difficult to organize resources effectively, minimize governance bloat, eliminate free-riders, and discover inefficiencies or bottlenecks in soft power.
Experimentation in using AI as an augmentation layer for DAOs are just beginning, but their potential impact is profound. The most common form is using highly trained LLMs to help direct labor capital within the DAO to effective causes, identify issues with proposals, and generally open up broader participation in both contributions and voting. There are also simpler tools like AwesomeQA which streamline DAO efficiency through search and automated response. Ultimately, we expect the ‘autonomous’ in DAO to become much more significant over time.
Autonomous Councils Voting Agents
Upstreet has taken multi-agent systems like those popularized by AutoGPT and applied them to their governance process as an early experiment. Each agent is defined by a sub-group of the DAO: artist, developer, BD strategist, PR, community manager, etc. These agents are then tasked with analyzing a proposal from a contributor and debate amongst each other the pros and cons. The agents then score its impact relative to their division and this score is aggregated. Human contributors can then evaluate their discussion and scoring before voting on an outcome, essentially offering diverse peer-review as a service.
This is particularly interesting since this process can surface aspects of a proposal that a human might miss, or enable humans to debate with an AI agent about its subsequent effects.
Autonolas uses an automation system to bring AI agent decisionmaking to smart contracts in an optimistic approach versus more computation heavy zk approaches. They released a novel experiment on governance which enabled any tokenholder to delegate their voting power within a DAO to an AI agent called the governatooor. Delegators could elect for the agent to vote with a moral affinity: good or evil. The service analyzes DAO forums for active votes and then executes the vote according to the user preference. While this is more of an experiment, it does highlight the possibility of AI agents trained with a user's preferences to actively participate and vote on their behalf.
Advanced Coordination Systems
MakerDAO has also talked at length of aiming for autonomous governance decisions with minimal human input. Their contribution to date has been an outline of Atlas - a hub containing all data relevant to Maker Governance that adapts in real-time. These units of data are organized in a Document Tree, providing context to prevent misunderstandings or misinterpretations. The Atlas will be in a JSON format and standardized for facilitating manipulation by AI and coding tools.
The Atlas will be used by various Governance AI Tools (GAITs) that participate in governance by automating interactions and prioritizing tasks for participants. Sample use cases include:
-
Project Bidding: GAITs can streamline the process for Ecosystem Actors to bid on projects by handling paperwork and ensuring proposals fit strategic goals.
-
Monitoring Rule Breaches: GAITs can assist in monitoring deliverables and rule adherence, flagging potential issues for human review.
-
Professional Advice Integration: GAITs can turn professional advice into formatted proposals, bridging the gap between governance and professional expertise.
-
Data Integration: GAITs allow for the easy integration of new data and experiences, helping the DAO to learn and adapt without repeating mistakes.
-
Language Inclusivity: GAITs can act as translators to make governance accessible in multiple languages, fostering a diverse and inclusive environment.
-
SubDAOs: The Atlas and GAITs can be applied to SubDAOs, allowing for experimentation and rapid development with the ability to learn from failures.
🧠 <> 🎮
One area of crypto x AI I am particularly excited about is in gaming. There are a multiple of novel game loops to be explored, such as procedural content games, generative virtual worlds, LLM-based narratives, cooperative games against or in conjunction with AI agents, and more.
While there are plenty of great examples in web2 of new game loops, here we will focus on web3-specific examples. However, it is worth mentioning the academic piece that woke up many to the possibility of multi-AI agent gaming environments. Researchers from Stanford and Google demonstrated this potential by applying LLMs to agents in a sandbox game environment. The LLM-powered agents exhibited impressive behavior, including independently spreading party invitations, forming friendships, asking each other out on dates, and coordinating to attend a party on time, all based on a single user-specified suggestion. The approach utilized an architecture that extended the LLM to store and synthesize memories into higher-level reflections, enabling dynamic behavior planning for the agents.
This research is a foundation for what is becoming the most explored - but still ripe for experimentation - game loop in web3 thus far. The core idea is how do we use AI agents in simulated environments with a high degree of autonomy or character and craft interesting and fun games around them.
Parallel Colony from the Parallel TCG team explores this concept by having AI agents gather resources and tokens in a game for the player. Using the ERC-6551 tokenbound standard, AI agents are NFT wallets that can transact in a game on behalf of a user. AI agents can create, mint, and store new in-game items, and also have personalities as defined by a fine-tuned LLM created by the team allowing them to have non-standard behaviors and traits that affect their actions in-game.
Most fascinating of the AI agent-based games conceptually though is Upstreet . Upstreet is a virtual world project that has some insanely creative functionality, such as an AI agents SDK, procedural questing, browser + VR, drag-and-drop interoperability, and social features in an environment called ‘The Street’ which will see players build their own experiences and interact within. Alongside players will be AI agents that developers (and players via a user friendly marketplace) can deploy with personalities and goals that impact the game environment. Most interesting of all is their research and development into the AI Director: an AI agent that decides on a goal such as “Skydive from the tallest building” or “Start a new religion” that users and agents participate in as a challenge. The Director then determines the winner when the round ends, rewarding players and agents with prizes, tokens, and NFTs. This could lead to very interesting and complex agent-player interactions, and we are remarkably excited to see the outcome, especially as this can directly result in high-value 3D environment research and data for even more advanced models in the future - something that OpenAI also seems to be interested in with their acquisition of an open source Minecraft style game .
Generative tools for creating virtual worlds is another area that enhances games. For example, Today has players design their own virtual islands and care for AI NPC companions. What’s particularly unique here is their use of generative creative tools for facilitating development of UGC in-game. Since the game is primarily based around these user-created islands, it is important to give players without 3D game development or art skills an opportunity to develop assets seamlessly. Arguably, a lot of the doldrums around metaverse-style gameplay is that content is lacking, which can be remediated with use of generative tools in the near-term.
AI agents need to be trained, and training itself can be an interesting game loop for players to explore. AI Arena offers a novel take on training AI agents, having players play a Super Smash Bros style game to slowly teach an AI agent for competitions via imitation training. Because the AI agent needs no breaks, it can infinitely play competitive tournaments for prizes against an always active competitor pool while a player fine tunes its playstyle async. This turns training into a game and enhances it with tokenomics.
Large scale cooperative game play by humans against a strong AI player is something that could be done in the past, but it is taken to new heights with the merger of tokenomics. Leela vs. the World from Modulus Labs is an experiment in this gameplay form. For this experiment, Modulus took the Leela chess engine and proved its outputs via a zk circuit. Players would then put up capital to vote on a human move to play against the AI, providing a creative prediction market around the outcome of the game. While proving times for the model were of course high given the state of zk at the time, it opened up the possibility of large-scale cooperation, esports-style prediction markets, and governance mechanisms for playing against a verifiable and sophisticated AI player.
Finally, fully onchain games, or autonomous worlds, will also be enhanced by AI. The most notable piece on the topic was Large Lore Models , which looked at using an LLM protocol layer to create ongoing lore that interops across moddable and interlinked game environments - player actions affect multiple game environments simultaneously in autonomous worlds and thus should carry with them higher dimensional lore for facilitating storylines. This is well-suited to an abstracted LLM layer built across multiple onchain games.
Infrastructure
AI x Crypto infrastructure warrants an entire piece unto itself, but here I’ll briefly go through some of the ideas we are seeing take shape.
Distributed Computing
To understand the need for cryptoeconomic systems for compute, its important to understand the core problem. GPU capacity is extremely bottlenecked to date, with the best available hardware such as the H100 seeing long wait-times extending up to a year out. This comes at a time where startups are raising huge rounds to acquire hardware, governments are scrambling to create their own supply for national defense purposes, and even the most well-funded teams like OpenAI having to pause feature releases because of limited compute capacity.
Many teams focused on decentralized computing and DePIN see an opportunity here: bootstrap permissionless clusters to service demand while providing crypto-incentives and minimal margins that make the networks highly competitive on pricing with web2 counterparts while simultaneously providing a better reward profile for hardware suppliers.
Machine learning can be broadly categorized into four main compute workloads:
-
Data pre-processing: Raw data is prepared and transformed into a usable format.
-
Training: ML models are trained on a large dataset to learn the patterns and relationships within the data.
-
Fine-tuning: ML models can be further optimized using a smaller dataset to improve the performance for a specific task.
-
Inference: The trained and fine-tuned models are run to make predictions.
We have seen the rise of more generalized compute networks like Render and Akash turn toward servicing more specialized compute like AI/ML. Render for example has tapped into providers building on top of their network like io.net to more directly serve AI customers, while suppliers like Akash have begun bringing onboard suppliers with in-demand hardware and exemplifying the power of the network via directly training their own models - the first example of this is a Stable Diffusion fork trained on only copyright-free materials. Livepeer is also specifically targeting AI video compute given their large network of orchestrators already servicing video transcoding use cases.
In addition, networks are now forming that target AI compute specifically, recognizing that core challenges around orchestration and verification can be solved more directly by building the entire chain or model around AI. Gensyn is one of the more notable examples of this, building a substrate-based L1 designed for parallelization and verification. The protocol uses parallelization to split larger computational workloads into tasks and pushes them asynchronously to the network. To solve the verification problem, Gensyn uses probabilistic proof-of-learning, a graph-based pinpoint protocol, and an incentive system based on staking and slashing. Although the Gensyn network is not yet live, the team predicts that the hourly cost for a V100-equivalent GPU on their network will be approximately $0.40.
Alternative training models exist as well, such as federated learning which has seen a revival in web3 after realizing blockchains can more properly incentivize these models. In short, federated learning is an approach where multiple parties train a model independently with updates regularly batched and sent to a global model. There are a variety of examples of this in practice, such as Google’s keyboard text prediction algorithm. Within web3, FedML and FLock are attempting to combine the federated learning approach with token incentives.
It’s also worth noting that decentralized data stores like Filecoin and Arweave , along with databases like Space and Time could play a significant role on the data pre-processing side.
Consensus-based ML
Another novel form of infrastructure around inference using blockchains is the concept of consensus-based ML. Bittensor is the most prominent example of this: a substrate-based L1 blockchain designed to improve machine learning efficiency and collaboration through its use of application-specific subnets. Each subnet has its own incentive system to service various use cases, from LLMs to prediction models to generative creativity. What’s unique about Bittensor is how it uses miners to coordinate quality outputs: Miners earn TAO, the native token, by providing intelligence via their ML models with outputs rated by verifiers. Since miners are incentivized for the best outputs, they consistently improve their models to remain competitive, resulting in a faster learning process coordinated by tokenomics.
The most recent exciting developments in the TAO ecosystem has been the Dynamic TAO proposal transitioning Bittensor to a more automated, market-driven mechanism design around token emissions and the Nous subnet launched to provide incentivized model fine-tuning in order to compete with the likes of OpenAI.
We are likely to see additional attempts at this type of system, where mining or consensus mediates model outputs in a way conducive to quality.
Intent is All You Need
Within DeFi, a recent narrative within the MEV space is around user intents and using economically-aligned solvers to execute those intents. The discussion of intents is often frenetic, but one thing has become increasingly clear: intents from users require higher-order semantic context to be parsed into executable code. LLMs could provide this semantic layer.
Propellerheads laid out the most articulate vision of using LLMs in the intents space to date here: https://www.propellerheads.xyz/blog/blockchain-and-llms .
In short, LLMs can help locate coincidences of wants (CoWs) opportunities by turning almost-matching intents into matching intents through semantic understanding. This can be done through inward intent renegotiation (e.g. "Is it ok to buy LUSD instead of USDC? I found a matching limit order and you'd save 0.3% on trading fees with this CoW.") and outward intent renegotiation (e.g. "I want to buy this other BAYC that you have; would you accept to sell that one for X ETH?").
There are other constructions that can be done here as well, which becomes especially interesting in the context of wallets and multisigs post-Account Abstraction. Projects like DAIN and Autonolas have experimented with using agents as signers on a wallet for both security and intent-based purposes for example. Talking to your wallet and having it execute txs on your behalf is not far off.
Also of note is the myriad of DeFi use cases, such as agent-based prediction markets, AI-managed economies, and ML-parameterized DeFi applications, check out my zkML piece for more ideas here.
Agent Economies
One of my favorite areas of infrastructure to date is in AI agent economies. There is a vision for the world in which everyone has their own agent, hires high quality and finely trained agents for services, or have autonomous agents fulfilling their objectives in a complex economy. In order to do this, it is vital that agents have a way to pay for and receive payments for their services. It is definitely possible that traditional fiat rails open up for these agents, but more likely is that agents will transact in crypto given its ease of use, speed of settlement, and permissionless nature.
Autonolas and DAIN are examples of projects working in this field. With Autonolas, agents are effectively nodes in a network working on a specific goal that are maintained by incentivized service operators, similar to a keeper network. These agents can be used for a variety of services, such as oracles, prediction markets, messaging, and more. DAIN takes a similar approach giving agents the ability to “discover, interact, transact, and collaborate with other agents from the network”.
Other Ideas
Outside of those above we also have also seen:
-
Decentralized vector databases for fine-tuning models like BagelDB
-
Wallets for API keys and SIWE for AI applications like Window.ai
-
Data provisioning services
-
Indexing and searching tools like Kaito
-
Block explorers and dashboards like Modulus Labs’ AI verification dashboard which right now is verifying a stream of inferences for the Upshot model
-
Development co-pilot like Dune’ s model for onchain SQL queries
-
Simulated agent testing environments
-
Bandwidth for data-scraping like the Grass Network
-
Synthetic data and human RLHF platforms
-
DeSci applications like the distributed bioML tools for protein-folding by LabDAO
A myriad of ideas are blossoming within web3 for servicing various areas of AI, so highlights are only provided here, but I strongly encourage exploring the projects above to get a sense of scale and utility.
The Intersection of it All
AI and crypto are synergistic. Both have leaned into open-source, pushed back against regulations, and are creating some of the largest wealth transfers in history. They need each other, each solving one another’s core challenges.
For crypto, AI solves issues in UX, facilitates more creative onchain use cases, enhances the ability of decentralized organizations and smart contracts, and unlocks true innovation at both the application and infrastructure layers.
For AI, crypto solves problems of authenticity and provenance, increases coordination around open-source models and datasets, helps with bootstrapping compute and data, and enables creators and agents to participate more directly in post-AI economics.
The challenge now is for crypto hackers, teams, and projects to understand this transition and embrace it. Creativity is now unbounded and we are sitting at the intersection of it all.
If you are interested in learning more about these intersections, come to our conference Convergence at ETHDenver.
If you are building within these intersections, DM me .
Disclaimer: The content of this article solely reflects the author's opinion and does not represent the platform in any capacity. This article is not intended to serve as a reference for making investment decisions.
You may also like
Texas court reverses Tornado Cash sanctions worth $455M
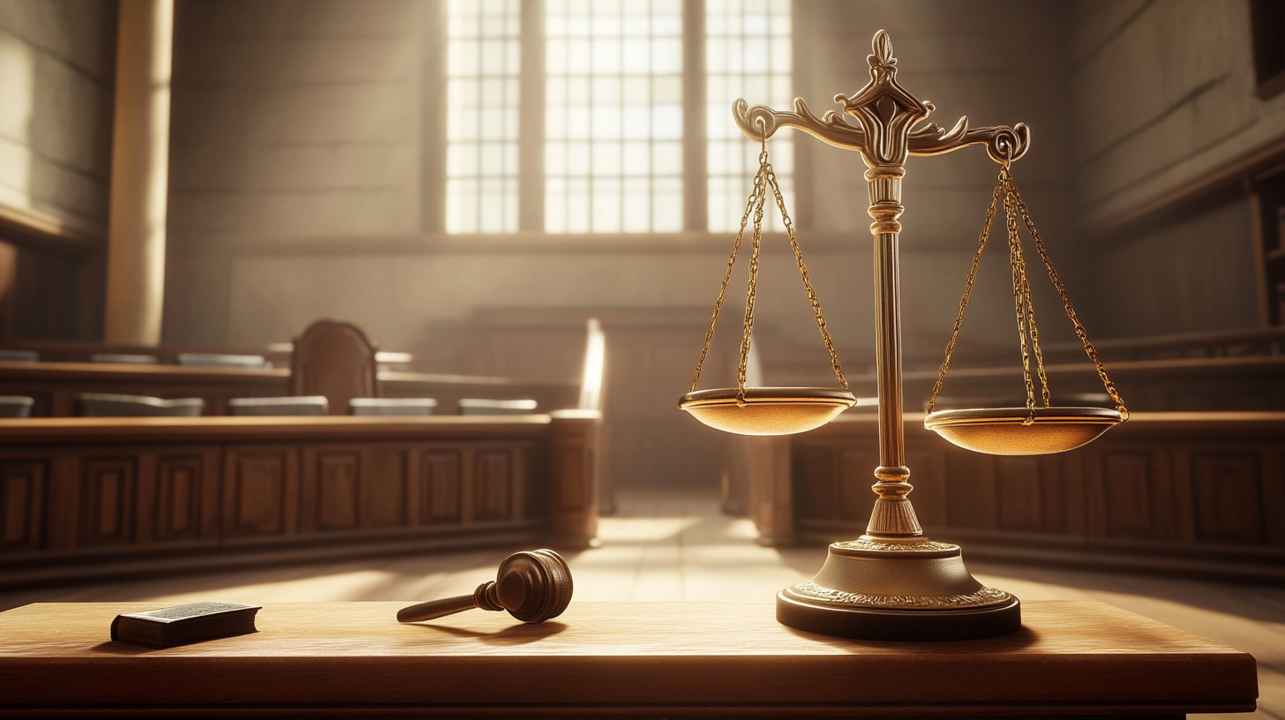
Calamos launches Bitcoin ETFs with capped returns and protection
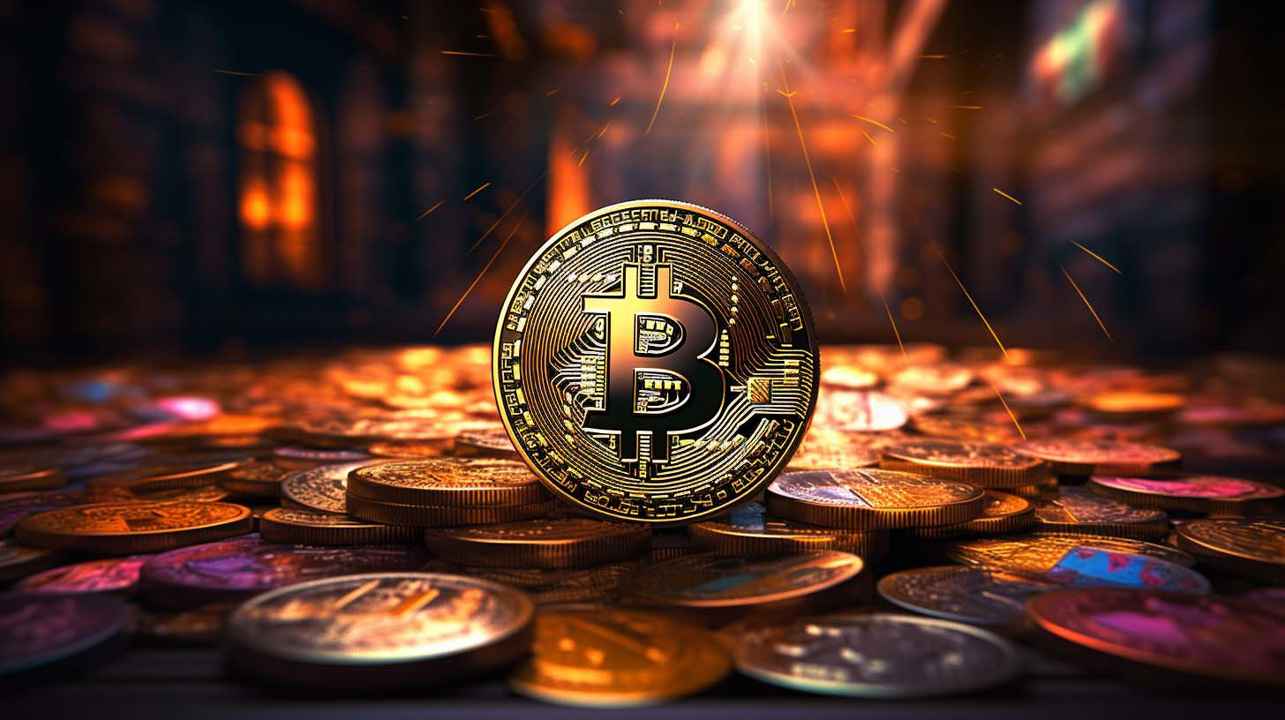
CME Group plans February 10 launch for Solana and XRP futures
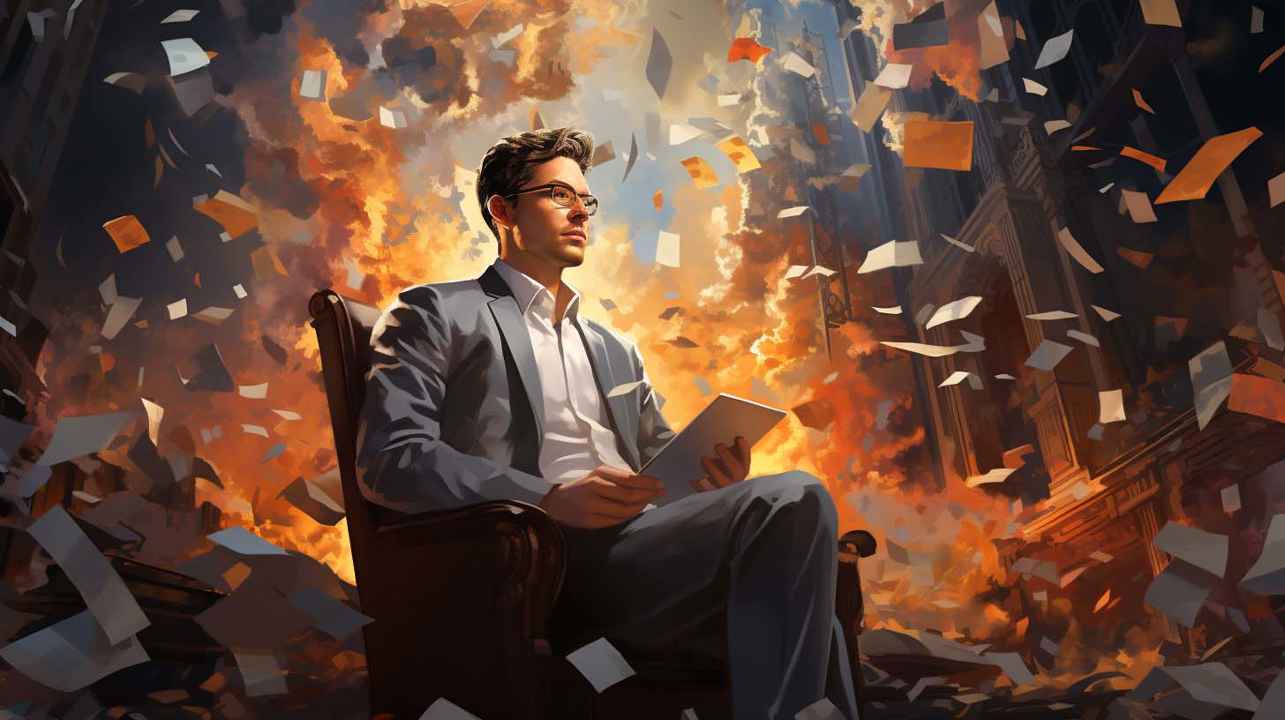
Ethereum developer Eric Conner leaves for AI after 11 years
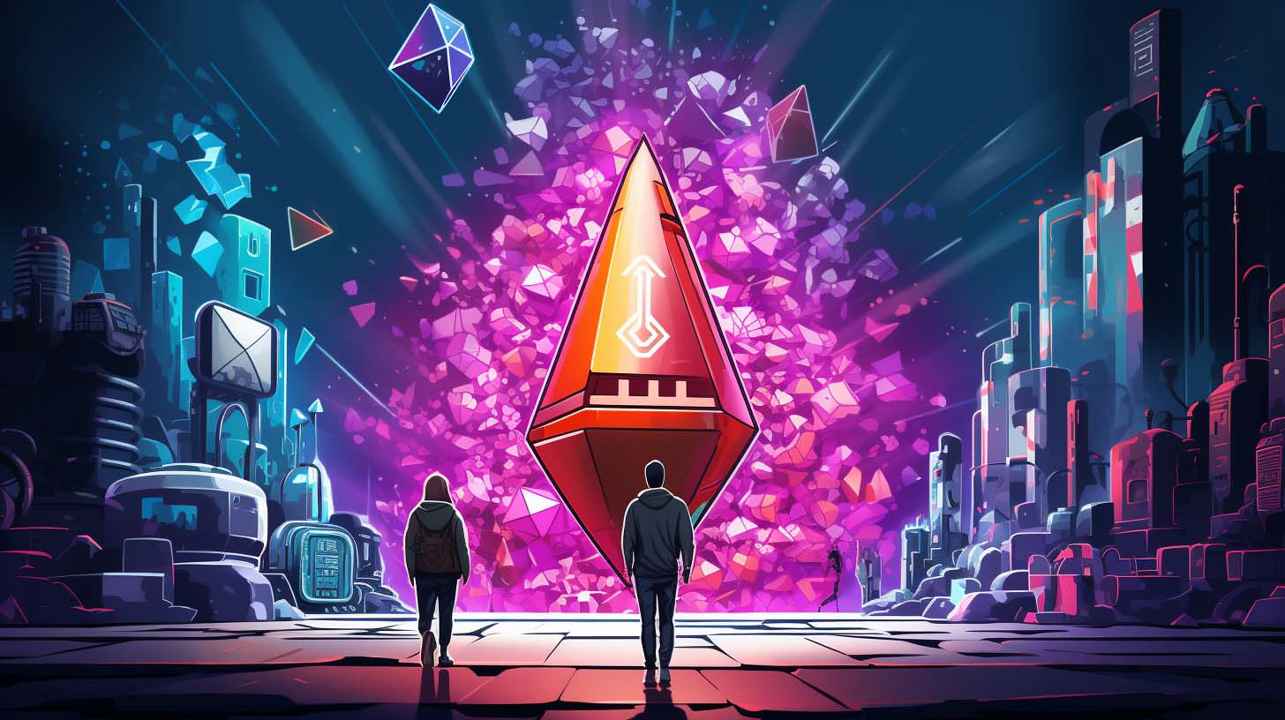
Trending news
MoreCrypto prices
More





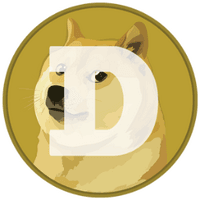


